South Australia Branch Meeting, September 2019
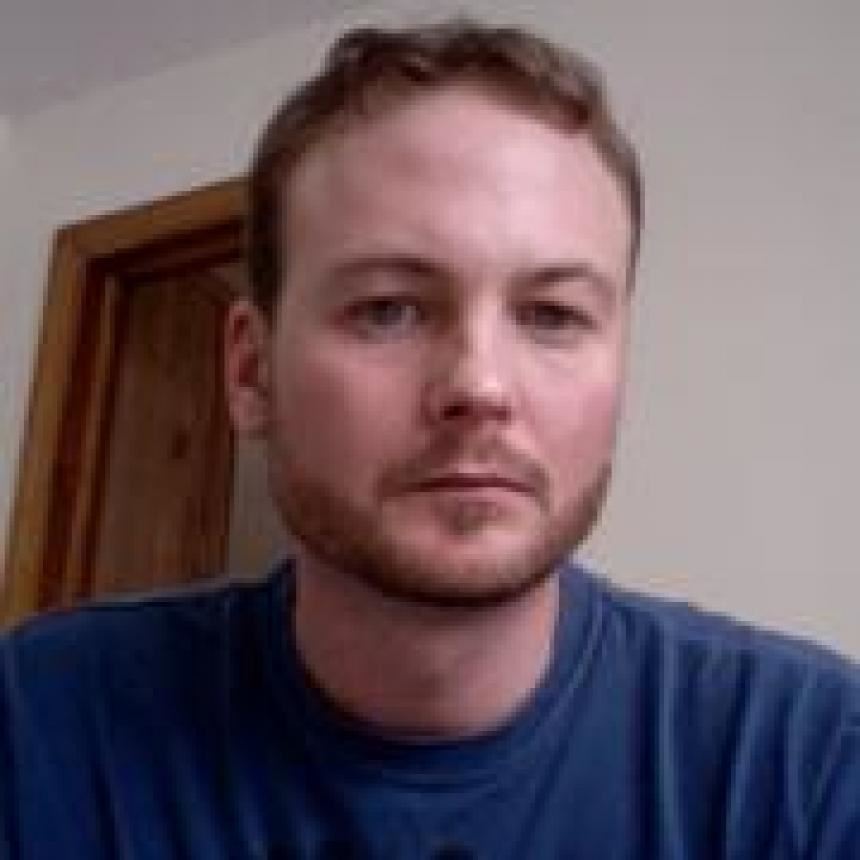
The speaker for the September meeting of the SA Branch was Peter Josef Kasprzak. The talk mainly discusses his master’s work at the Biometry Hub, Adelaide University which looks at the use of sampling methods in agriculture trials. The ultimate aim is to make robust sampling methods available to end users such as farmers who may have little computational experience. However, first there has to be a proof of concept for a semi-automated sampling protocol in a typical agricultural scenario. The motivating scenario was a seed trial where the emergence of faba bean seedlings was estimated. Automatic data collection using drones was explored and computer vision and machine learning techniques for image processing was compared in a sampling context.
A data collection protocol for sampling is not always conducted in agricultural experiments. An exploration of the literature found that often papers offered no justification for sample sizes used. This exacerbates the issue of non-reproducibility of experiments highlighted by Baker (2016) where more than 70% of researchers failed to reproduce another scientists experiments and 50% failed to reproduce their own. It’s clear that an unbiased random sampling protocol is needed to ensure good study design.
Mcintyre (1952) introduced the ranking set sampling (RSS) method (without theoretical justification). RSS compares the units before the final selection which increases the structure on the measured data, without analysis of all the sampled units (Ozturk and Wolfe, 2000). In common agriculture distributions RSS performs better than other sampling methods for example simple random sampling SRS. Takahasi and Wakimoto (1968) showed the theory behind RSS showing the variance of RSS will always be less than SRS. RSS has the advantage that ranking can be done with auxiliary variable which are highly correlated and easily gathered variables. While the infinite paradigm of RSS is well mapped the finite paradigm is not.
In a field trial scenario the sampling paradigm is finite. In finite sampling there are 3 different levels of replacement. No items returned, all items returned and all items returned except for the selected unit. Simulation is an obvious tool to determine what sort and level of sampling should be undertaken. R Shiny was used to create a web based app which performs a simulation study based on different sampling protocol. Simply load in some data and simulations can be run in real time which robustly select the best sampling protocol. The app outputs a csv file with positions in the field to be sampled.
So how can this be implemented in the faba bean emergence trial? A drone was used to collect images of a field where faba bean seedlings were emerging at the plot level. Manual and automated flights were undertaken with image stitching of footage needed before processing for prediction of individual seedlings could be undertaken. Microsoft ICE and webODM, both free programs, used to stitch the smaller images taken by the drone together to form a composite image were compared. webODM proved to be a superior program for handling changing conditions, but required an intermediate level of computing knowledge to use. ICE was very simple to use, but was far more susceptible to changing conditions, and blurred joins in the final composite. With the final composite image, two approaches for processing the image and predicting the seedling numbers were compared-Computer Vision (CV) and Neural Networks (NN). Python programs for CV and NN were used to obtain an estimate per pre-specified grid area in the field, which was then used in the sampling app as a ranking variable for the RSS protocol. The study found that CV is easier to use but NN were superior as long as there is an adequate training set size which is diverse. In CV there are limited options to deal with false positives in high level stubble and potential over fitting can occur in NN with a greater level of work required to create the training data set. Issues such as seedling stress, nutrient and water stress effect the colours that you get in CV but NN can sort this out. An ideal solution is to use both in conjunction- start with CV to propose positive candidates and then use NN to disqualify false positives to leave only true hits to improve ranking.
The Shiny app sampling coordinates can be matched to the photographic coordinates. This means that images collected and processing by NN can be mapped to sampling coordinate position. Pete presented results from the faba bean study which compared different finite sampling scenarios under SRS and RSS. The results showed that RSS was superior across all finite sampling replacement scenarios with relative efficiencies (variance SRS/variance RSS *100) between 143.1-216.1.
McIntyre, G. A. (1952). A Method for Unbiased Selective Sampling,Using Ranked Sets. Australian Journal of Agricultural Research,(3):385-390.
Ozturk, O. and Wolfe, D. A. (2000). Optimal allocation procedure in ranked set sampling for unimodal and multi-modal distributions. Environmental and Ecological Statistics, 7(4):343-356.
Takahasi, K. and Wakimoto, K. (1968). On unbiased estimates of the population mean based on the sample strati_ed by means of ordering. Annals of the Institute of Statistical Mathematics, 20(1):1-31.
The detail of his talk can be found by contacting peter.kasprzak@adelaide.edu.au. A dinner was held right after the meeting at Sukhumvit Soi 38, 54 Pulteney Street, Adelaide.
By Helena Oakey