MEL/SYD/CBR time via online
Rates of kidney cancer have been increasing, with localized small incidental tumors experiencing the fastest growth rates.
Brian Egleston, Ph.D. will discus how much of the increase could be due to the increased use of CT scans, MRIs, and ultrasounds for conditions unrelated to kidney cancer. Many of these tumors might never have been detected or become symptomatic in the past. This suggests that many patients might benefit from less aggressive therapy, such as partial rather than total removal of the kidney. It is even possible that some patients do not need treatment for localized kidney cancer.
In this work, we propose using a principal stratification framework to estimate the proportion and characteristics of individuals who have large or small hazard rates of death in two treatment arms. This allows us to predict who might be helped or harmed by aggressive treatment. We use Weibull mixture models as part of the project. This work differs from much previous work in that the survival classes upon which principal stratification is based are latent variables. That is, survival class is not a variable observed in the data. We apply this work to estimate surgical treatment effects using linked U.S.A. Surveillance Epidemiology and End Results-Medicare claims data.
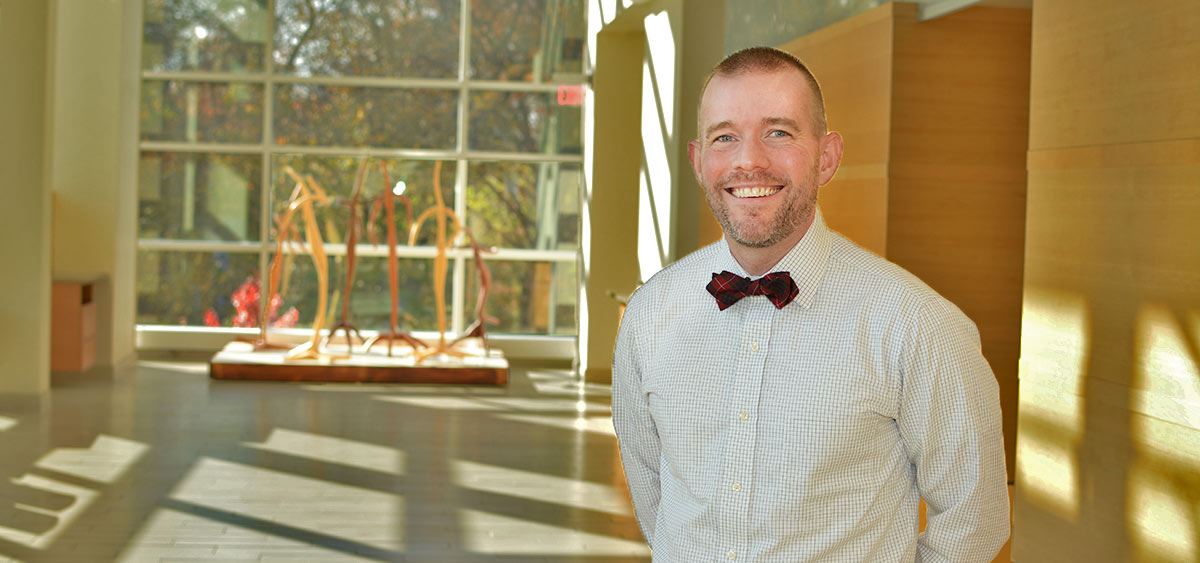
Brian Egleston, Ph.D. is an Associate Research Professor and Biostatistician at the Fox Chase Cancer Center in Philadelphia, USA. The Center is part of the Temple University Health System. He has had papers published in both the methodological literature (e.g. Journal of the American Statistical Association, Biometrics) and the substantive literature (e.g. New England Journal of Medicine). He is actively involved in a number of behavioral clinical trials, and has experience accounting for missing data in such a context. He is specifically trained in the statistical field of causal inference, and many of his publications are related to methods for the analysis of observational data when confounded by comorbidities or other variables is of concern. More recently, he has become involved in examining the statistical properties of natural language processing algorithms.
This event is free for SSA members,but you do need to register.